In various social and economic contexts, individuals often adapt their decisions and outlooks in response to group norms and the behaviour of their peers. This tendency is commonly termed “peer effects” or “endogenous social effects.” While it is intuitive to observe that a household’s decisions about, say, resource allocation or schooling may be shaped by neighbours or colleagues, understanding the precise channels of influence can be much more complex. At the heart of this complexity is the reflection problem, a term I find useful in explaining why it is often challenging to distinguish between the influence peers exert on an individual and the parallel influence that the individual’s traits might have on their peers and environment.
This article provides a structured exploration of the reflection problem, emphasising how it arises and why it remains a persistent issue in econometric research. Drawing upon existing scholarship in social interactions and peer effects, I illustrate how underidentification can easily occur if one attempts to measure the impact of group averages on individual outcomes without introducing additional assumptions or well-chosen instruments. In practical terms, this challenge has direct implications for how researchers and policymakers design interventions, interpret correlations, and assess the likely efficacy of group-based policy strategies. By the end, I hope to highlight both the magnitude of the reflection problem and the pathways through which it can be mitigated.
The notion that people’s outcomes are interlinked is not new. Scholars in sociology, anthropology, and economics have long recognised that social norms, group pressures, and community contexts play pivotal roles in shaping individual behaviour. Early sociological works placed great emphasis on social structures, while economists historically favoured models centred on individual rational choice. Over time, however, there has been a growing appreciation that ignoring social dynamics can lead to incomplete explanations of economic and social phenomena.
Charles F. Manski’s (1993) seminal paper on the reflection problem crystallised the challenge of empirically measuring peer effects. He argued that when one attempts to regress an individual’s outcome on the average outcome of their group, one faces a conundrum: how do we know if the group’s average outcome is truly influencing the individual, or if it merely reflects the aggregation of individual outcomes—potentially including that of the individual in question? Additionally, how do we separate the effect of group-level attributes (contextual effects) from genuine spillovers (endogenous effects)? The reflection problem is thus at the heart of distinguishing between correlation and causation in social interactions.
A common way to formalise peer effects is through a linear model. Suppose \(y_i\) is the outcome for individual \(i\) in group \(g\). Let \(\bar{y}_g\) denote the group’s average outcome, \(\bar{z}_g\) the group’s average characteristics, and \(z_i\) the individual’s own characteristics. A simple specification is:
\( y_i = \alpha + \beta \bar{y}_g + \gamma \bar{z}_g + \delta z_i + \epsilon_i. \)
Here, \(\beta\) captures the endogenous peer effect, \(\gamma\) captures the contextual or exogenous group effect, and \(\delta\) measures how much individual attributes directly affect the outcome. At first glance, this model seems straightforward. However, the challenge arises because \(\bar{y}_g\) is itself a function of the outcomes \(y_j\) for all individuals \(j\in g\), including \(y_i\). This simultaneity means that a naive regression of \(y_i\) on \(\bar{y}_g\) risks conflating the individual’s effect on the group with the group’s effect on the individual.
The reflection problem arises because group outcomes and individual outcomes often mirror each other. If you aggregate the model across the group, you get:
\( \bar{y}_g = \frac{1}{N_g} \sum_{j \in g} y_j. \)
Substituting each \(y_j\) from the individual-level equation into \(\bar{y}_g\) introduces a circularity: \(\bar{y}_g\) appears on both sides of the equation, entangling the roles of \(\beta\), \(\gamma\), and \(\delta\).
\( \bar{y}_g = \alpha + \beta \bar{y}_g + (\gamma + \delta) \bar{z}_g + \bar{\epsilon}_g \nonumber \)
\( \bar{y}_g(1 - \beta) = \alpha + (\gamma + \delta)\bar{z}_g + \bar{\epsilon}_g \nonumber \)
\( \bar{y}_g = \frac{\alpha}{1 - \beta} + \frac{\gamma + \delta}{1 - \beta}\bar{z}_g + \frac{\bar{\epsilon}_g}{1 - \beta} \)
After solving for \(\bar{y}_g\) and plugging it back into the individual equation:
\( y_i = \alpha + \beta\left(\frac{\alpha}{1 - \beta} + \frac{\gamma + \delta}{1 - \beta}\bar{z}_g\right) + \gamma \bar{z}_g + \delta z_i + \epsilon_i \)
\(= \frac{\alpha}{1 - \beta} + \left(\frac{\beta(\gamma + \delta)}{1 - \beta} + \gamma\right)\bar{z}_g + \delta z_i + \epsilon_i + \frac{\beta\bar{\epsilon}_g}{1 - \beta} \)
Thus, you typically end up with a reduced form that reveals you can only identify certain linear combinations of the parameters, not each one independently:
\( y_i = \underbrace{\frac{\alpha}{1 - \beta}}_{\text{Intercept}} + \underbrace{\left(\frac{\gamma + \beta\delta}{1 - \beta}\right)}_{\Pi}\bar{z}_g + \delta z_i + u_i \)
Where: \( \Pi = \frac{\gamma + \beta\delta}{1 - \beta} \)
This is the essence of underidentification.
In more intuitive terms, if the average group outcome is partly driven by each individual’s outcome, it is not clear whether changes in the group outcome cause changes in the individual or vice versa. This simultaneity is precisely what Manski highlights. Without additional information or constraints, one cannot simply look at a correlation between an individual’s outcome and the group’s outcome and conclude that peer influence is at work.
Econometric identification is about whether one can isolate unique values for parameters of interest from the data. In the reflection problem, there are three structural parameters—\(\beta\), \(\gamma\), and \(\delta\)—but fewer degrees of freedom in the reduced form (only two estimable coefficients (\(\Pi, \delta\))), leading to underidentification. Consequently, naive OLS regressions that include \(\bar{y}_g\) as an explanatory variable risk mixing up these effects, resulting in biased or inconsistent estimates.
This underidentification matters greatly in practice. Suppose you observe that in a certain region, both the group average outcome and individual outcomes are high. One might be tempted to attribute this to strong peer effects. However, it could be that the region simply has superior infrastructure or a well-designed policy environment that raises everyone’s outcome, creating an illusion of peer influence. If policymakers interpret this correlation as evidence of strong peer effects and design interventions accordingly, they may be disappointed when those interventions fail to replicate the observed outcomes in other contexts.
As Manski (1993) and subsequent researchers have noted, solving the reflection problem typically requires additional structure or data. Below are some of the most common strategies:
- Exclusion Restrictions: One might assume that certain variables do not appear in the individual’s own outcome equation, or that individual-level characteristics do not enter into the group outcome equation. For instance, setting \(\delta=0\) can help disentangle the parameters. However, such assumptions must be well justified and may not always be realistic.
- Instrumental Variables (IV): Another approach is to find variables that influence \(\bar{y}_g\) but are exogenous to \(y_i\). If a credible instrument can be identified—perhaps a policy that randomly assigns resources to certain groups but not others—this exogenous variation can help isolate the true effect of the group outcome on the individual. The difficulty lies in identifying a strong and valid instrument that meets the necessary exogeneity criteria.
- Random Group Assignment: In some controlled experiments, individuals are randomly assigned to groups. If the composition of groups is random, then variations in \(\bar{y}_g\) can be seen as exogenous to individual traits. This strategy is often employed in educational research where students are randomly assigned to dormitories or classrooms, making it easier to attribute differences in individual outcomes to the group environment rather than self-selection.
- Network Analysis: Instead of treating an entire class or neighbourhood as a single group, one might exploit detailed data on who interacts with whom. By mapping out social networks, researchers can attempt to identify exogenous changes in specific ties, potentially overcoming some of the reflection problem’s confounding factors.
Each of these methods has strengths and weaknesses. Exclusion restrictions may be too strong; finding valid instruments can be difficult; random assignment is not always feasible; and network data may not be available. Nonetheless, these strategies highlight the creativity researchers have applied to circumvent underidentification.
Beyond these classical approaches, partial population experiments and quasi-experimental designs also show promise. In some public health interventions, for instance, only a fraction of individuals in a community receive a treatment. By comparing treated and untreated individuals within the same environment, one can sometimes tease out whether changes in the group outcome truly cause changes in individual outcomes.
Empirical examples abound. In education, random assignment to roommates has been used to study how peer academic performance affects individual grades. In labour economics, the employment status of neighbours has been investigated to see if it influences an individual’s job search behaviour. In health, researchers have explored whether obesity or smoking “spread” within social networks. In each of these contexts, the reflection problem can obscure the distinction between genuine peer influence and correlated environmental factors unless carefully addressed.
The reflection problem persists because social interactions are inherently bidirectional: people influence their peers, who in turn influence them back. This mutual causation can look like a mirror, reflecting each individual’s behaviour in the collective outcome. Without an exogenous shock, random assignment, or strong assumptions, we cannot easily separate who is influencing whom.
Moreover, group formation is often endogenous. Individuals may select into certain schools, neighbourhoods, or social circles based on unobserved traits, further complicating identification. If the same unobserved trait drives both group membership and outcomes, naive analysis will confound peer effects with selection effects. A randomised approach or a natural experiment can mitigate this problem but is rarely available outside controlled settings.
In response to these challenges, more advanced econometric techniques have emerged. Some researchers employ simultaneous equations models that explicitly account for the fact that \(y_i\) and \(\bar{y}_g\) are determined together. Others use Bayesian hierarchical models or structural approaches that specify the nature of social interactions more precisely. While these methods can be powerful, they often require detailed data and rely on assumptions that might not always hold in real-world settings.
Another promising direction involves leveraging big data and network science. If one has a granular map of social ties, one might detect exogenous changes in certain parts of the network and observe how they ripple through connections. However, collecting and analysing such data can be resource-intensive and may raise privacy concerns.
Accurately identifying peer effects is not just an academic exercise; it has profound policy implications. In education, policymakers often group students in hopes of boosting performance through peer influence. In agriculture, interventions may rely on “lead farmers” to demonstrate new techniques, expecting the rest of the group to follow. In public health, partial vaccinations or community health drives may hinge on the assumption that those who benefit will encourage others to do the same. If peer effects are overestimated due to the reflection problem, these policies may yield disappointing results. Conversely, if peer effects are underestimated, we may miss opportunities for collective interventions that leverage social influence effectively.
Some critics argue that while the reflection problem is conceptually significant, in practice, partial or natural experiments often exist that can shed light on genuine peer effects. Others contend that many observed correlations in group data might be spurious unless one can fully account for all relevant confounders. This debate underscores the tension between the complexity of real-world social interactions and the simplifying assumptions often required for tractable econometric models.
Ultimately, the reflection problem remains a useful reminder that correlation—especially within social groups—does not automatically imply causation. It pushes researchers to be more rigorous in their identification strategies, either by designing experiments that circumvent simultaneity or by applying robust econometric methods that can separate endogenous effects from contextual and correlated factors.
- Workplace Productivity: In an open-plan office, it may look like employees influence one another’s productivity, but a high-performing office may simply reflect strong organisational culture or management practices.
- Adolescent Behaviour: Teenagers might appear to emulate their peers’ choices regarding substance use, but they may also be responding to the same environmental factors, such as community norms or family background.
- Technology Adoption: People often adopt new gadgets or platforms if they see friends doing so, yet the group’s high adoption rate might reflect shared demographic traits rather than genuine social contagion.
- Examine Group Formation: Determine whether group membership is random, policy-driven, or self-selected. Understanding how groups form can reveal potential sources of bias.
- Look for Exogenous Variation: Seek out natural experiments, policy changes, or random assignment that create variation in group averages unrelated to individual traits.
- Consider Instrumental Variables: Identify plausible instruments that shift \(\bar{y}_g\) but do not directly affect \(y_i\). Evaluate the validity of these instruments rigorously.
- Use Network Data if Possible: Detailed network information can help pinpoint which peers truly matter and how influence propagates through social ties.
- Conduct Robustness Checks: Try different specifications, subsamples, and control variables to ensure results are not driven by unobserved confounders.
Despite methodological advances, the reflection problem remains a central concern in social science research. With more detailed datasets and computational methods, we are better equipped than ever to tackle complex interactions. Yet, the fundamental simultaneity at the core of peer effects means that perfect identification may remain elusive unless the data or experimental design is particularly fortuitous.
Future research may focus on integrating machine learning techniques with traditional econometrics, exploring how large-scale network data can be harnessed to tease out exogenous shocks. Additionally, more interdisciplinary work that combines economic models with sociological or psychological insights could lead to richer theories of how individuals and groups mutually shape each other’s behaviour.
The reflection problem stands as a powerful reminder that correlation does not imply causation, especially in social contexts where people shape and are shaped by their peers. While Manski’s original articulation focused on the theoretical challenge of underidentification, decades of subsequent research have confirmed the practical difficulties of pinning down peer effects without strong assumptions or exogenous variation. Nonetheless, the importance of peer influence in fields as diverse as education, health, and agriculture means that overcoming the reflection problem is vital for both academic research and effective policy design.
Researchers can take heart that numerous creative solutions—ranging from carefully chosen exclusion restrictions to randomised experiments—have proven successful in many cases. Even so, caution is warranted whenever strong claims about peer effects are made without credible identification strategies. By remaining mindful of the reflection problem and its implications, economists and other social scientists can continue refining our understanding of how group dynamics inform individual choices, ultimately leading to more nuanced and impactful interventions.
Manski, C. F. (1993). “Identification of Endogenous Social Effects: The Reflection Problem.” Review of Economic Studies, 60(3), 531–542.
Brock, W. A., & Durlauf, S. N. (2001). “Discrete Choice with Social Interactions.” Review of Economic Studies, 68(2), 235–260.
Sacerdote, B. (2001). “Peer Effects in Education: Evidence from College Roommates.” Quarterly Journal of Economics, 116(2), 681–704.
Angrist, J. D., & Pischke, J.-S. (2009). Mostly Harmless Econometrics. Princeton University Press.
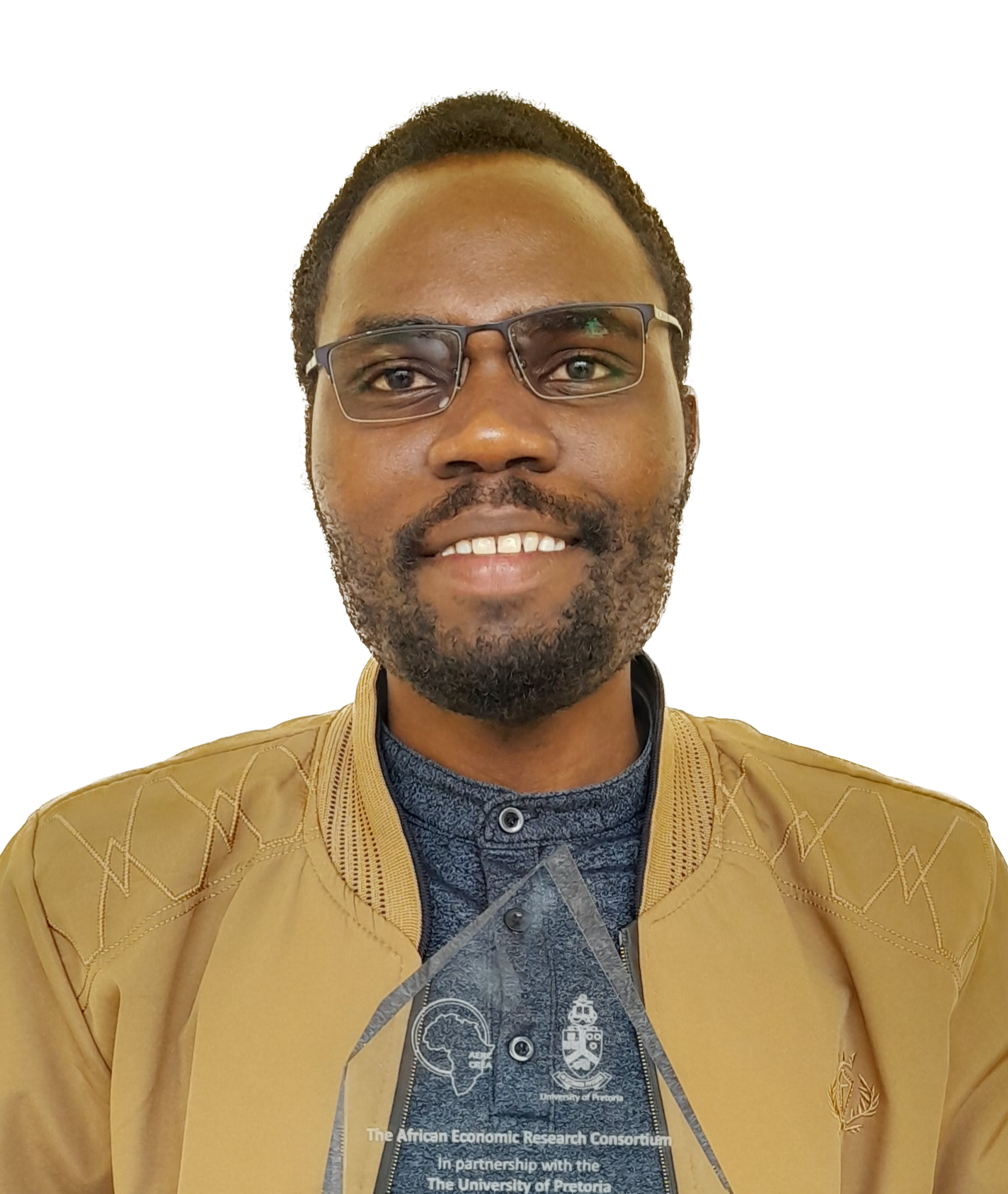