Causal inference represents one of the most challenging pursuits in empirical research—a true intellectual frontier where statistical methods and substantive domain knowledge converge. Questions such as “Does a novel policy enhance productivity?” or “Can public health interventions measurably reduce morbidity?” require not only robust data but also rigorous, context-aware analytical frameworks.
This post examines the inherent limitations of what some consider “shortcut” methodologies—like propensity score matching or sensitivity analyses (E-values)—and explains why no single method can provide an infallible answer. Instead, it argues for a comprehensive, thoughtful approach to causal inference that synthesizes diverse techniques and deep subject-matter expertise.
The Complexity of Observational DataUnlike controlled experiments, observational data are by nature messy and imbued with real-world complexities. Researchers seldom have the luxury of random assignment, and as a result, the data are vulnerable to several validity threats:
- Selection Bias: The characteristics of individuals or units that receive a treatment may systematically differ from those that do not, thereby skewing the observed effect.
- Confounding Factors: Unmeasured variables may simultaneously influence both the treatment and the outcome, obscuring the true causal relationship.
- Reverse Causality: The possibility that the outcome may, in turn, affect the treatment cannot be ruled out in observational settings.
As a consequence, no statistical adjustment—be it regression analysis or propensity score matching—can completely neutralize these inherent biases without careful consideration of underlying assumptions and external evidence.
Limitations of Shortcut MethodsIt is a common misconception that complex statistical procedures alone can resolve the challenges of observational research. For example:
- Propensity Score Matching: Although it balances observed covariates across groups, it does not remedy bias stemming from unobserved confounders.
- Sensitivity Analysis (E-values): This tool quantifies how robust an effect might be to hidden bias, yet it does not confirm the absence or magnitude of such confounding influences.
- Automated Machine Learning Algorithms: While these methods enhance model selection and prediction, they remain agnostic to the nuanced causal mechanisms at work and cannot substitute for domain expertise.
In essence, reliance on any one of these approaches without a thorough theoretical and contextual examination is akin to expecting a “magic bullet” to deliver definitive causal insights.
Difference-in-Differences (DiD) as an Analytical FrameworkThe Difference-in-Differences (DiD) methodology is one of the most widely used quasi-experimental approaches in observational studies. DiD capitalizes on temporal variations by comparing outcome changes in a treatment group to those in a control group over time. The underlying assumption is that, in the absence of the intervention, both groups would have evolved in parallel.
However, the credibility of DiD estimates rests on several critical assumptions:
- Parallel Trends: Absent the intervention, the evolution of outcomes in both groups would be similar. Divergence in pre-treatment trends can invalidate this assumption.
- No Anticipation: Subjects must not alter their behavior in expectation of the treatment, as pre-treatment adjustments can contaminate the estimated effect.
- Stable Unit Treatment Value Assumption (SUTVA): The treatment applied to one unit should not affect the outcomes of another, ensuring that the observed changes can be solely attributed to the intervention.
When these assumptions hold, DiD can provide compelling evidence regarding causal effects. Nonetheless, its application demands rigorous verification through pre-trend analyses, robustness checks, and careful contextual interpretation.
Even with an ostensibly robust DiD design, researchers must remain vigilant about several practical challenges:
- Verification of Parallel Trends: Pre-treatment data should be scrutinized to ensure that both groups follow similar trajectories before the intervention.
- Impact of External Shocks: Unrelated events, such as economic downturns or public health crises, can differentially affect treatment and control groups, thereby confounding causal interpretations.
- Potential Spillover Effects: When the intervention indirectly influences control units (for example, through regional economic linkages), the estimated effect may be diluted or biased.
- Substantive vs. Statistical Significance: Even statistically significant results must be interpreted in light of their practical implications, ensuring that the magnitude of the effect is both meaningful and plausible.
- Robustness and Sensitivity: Complementary analyses—such as event studies, placebo tests, and alternative model specifications—are essential to build confidence in the causal claims.
In the realm of observational studies, no single method or shortcut can substitute for a meticulous, context-driven analysis. The process of causal inference remains an iterative dialogue between data, theory, and substantive expertise.
The Imperative of Rigorous Scientific Judgment
In conclusion, while advanced statistical methods like DiD and propensity score matching offer valuable tools for causal analysis, they are not panaceas. Rather, they must be embedded within a broader framework of critical inquiry—one that rigorously interrogates assumptions, integrates domain knowledge, and continuously refines its theoretical underpinnings.
As scholars and practitioners, we must acknowledge that causal inference in observational research is as much an art as it is a science. There is no “magic bullet” that can supplant the need for thoughtful, context-aware analysis. Instead, a multi-faceted approach—grounded in robust methodology and enriched by substantive expertise—remains the most reliable path to uncovering causal truths.
- Angrist, J. D., & Pischke, J.-S. (2009). Mostly Harmless Econometrics: An Empiricist’s Companion.
- Imbens, G. W., & Rubin, D. B. (2015). Causal Inference for Statistics, Social, and Biomedical Sciences.
- Callaway, B., & Sant’Anna, P. H. (2021). “Difference-in-Differences with Multiple Time Periods and an Application on the Minimum Wage.” Journal of Econometrics.
- Cole, S. R., & Hernán, M. A. (2002). “Constructing Inverse Probability Weights for Marginal Structural Models.” American Journal of Epidemiology.
Author’s Note
When implementing quasi-experimental methods like DiD, it is imperative to contextualize your findings within the broader framework of your research. Engage with domain experts, perform rigorous robustness checks, and never lose sight of the underlying assumptions. Remember: methodologies are tools to aid scientific inquiry—not substitutes for careful reasoning and critical analysis.
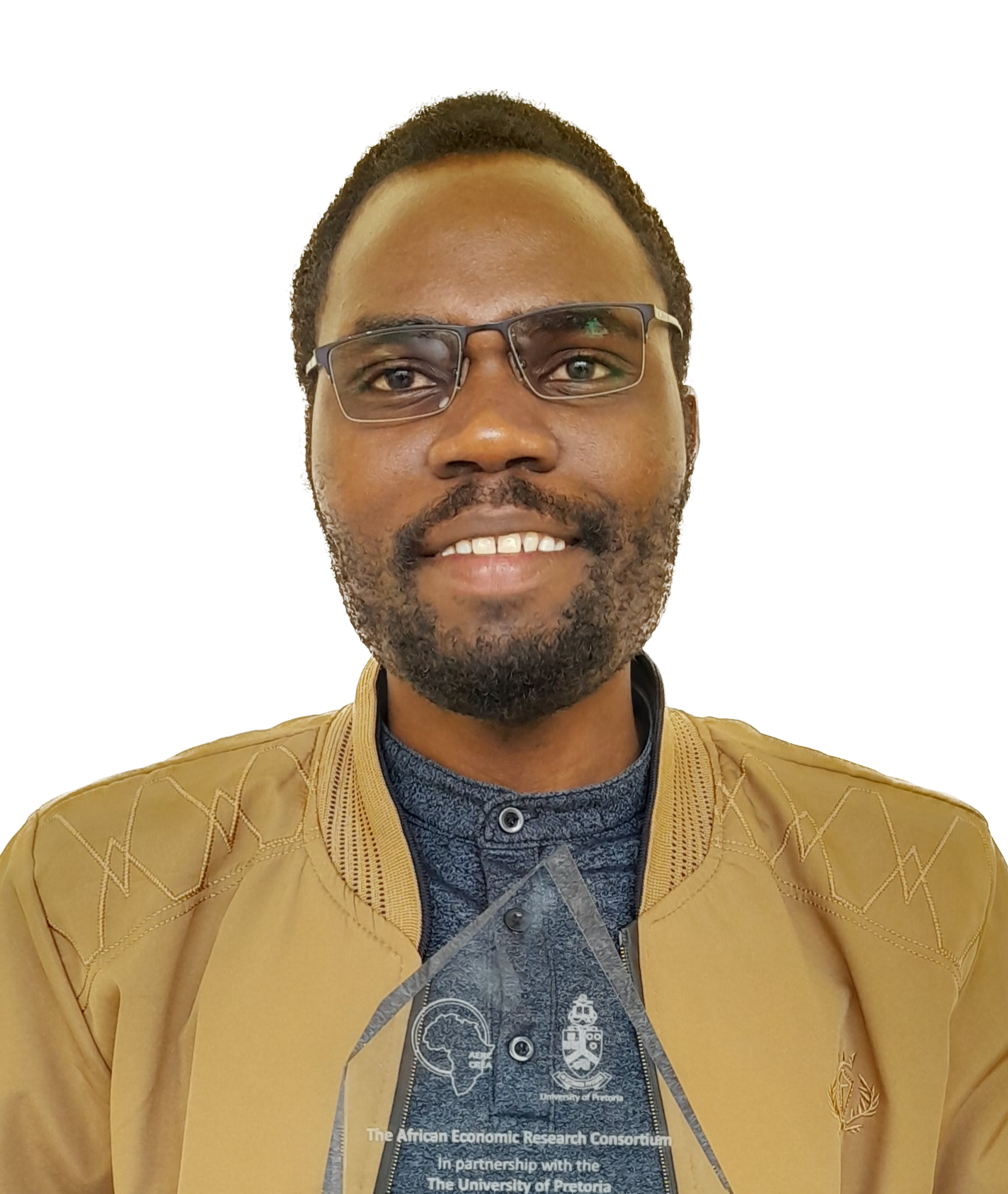